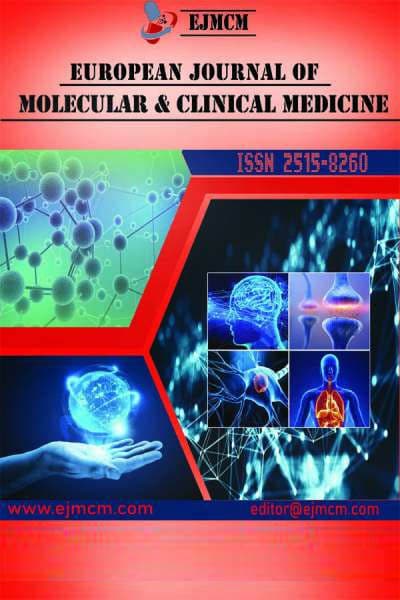
Chief Editor Name
C. C. Yin
Associate Professor,Cancer Center, USA
Publisher:
EJMCM, International House
The European Journal of Molecular and Clinical Medicine is indexed by Web of Science ESCI (discontinued December 2022), CrossRef, JISC KB+, SHERPA RoMEO, Cengage Learning, and Embase (discontinued July 2023) and is available as an open-access journal on the Internet. The European Journal of Molecular & Clinical Medicine was included in Scopus in 2018 and 2020. Full-length articles and abstracts are available online at Scopus. The European Journal of Molecular and Clinical Medicine is an open-access journal. Interested readers can view, download, print, and redistribute our articles without a subscription, which guarantees that the author.
Our Indexing Partners
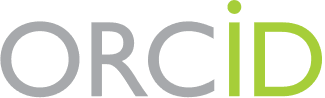
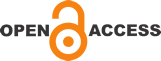
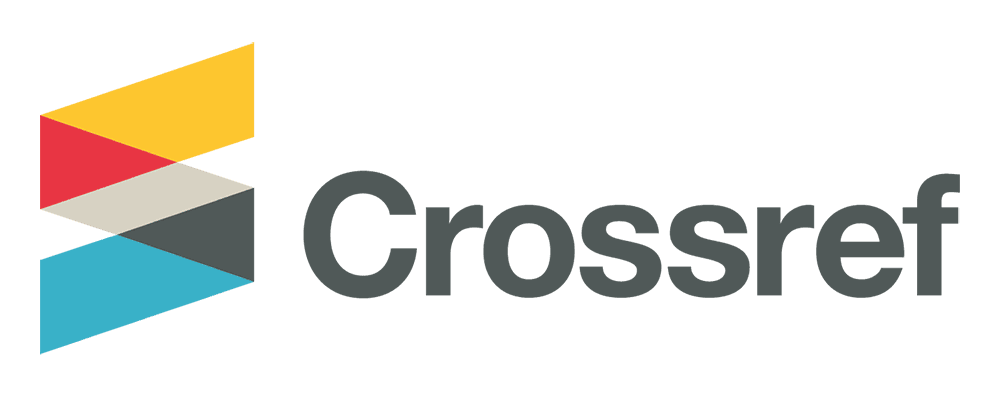
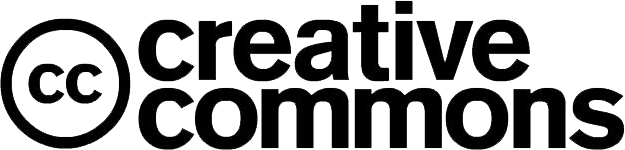